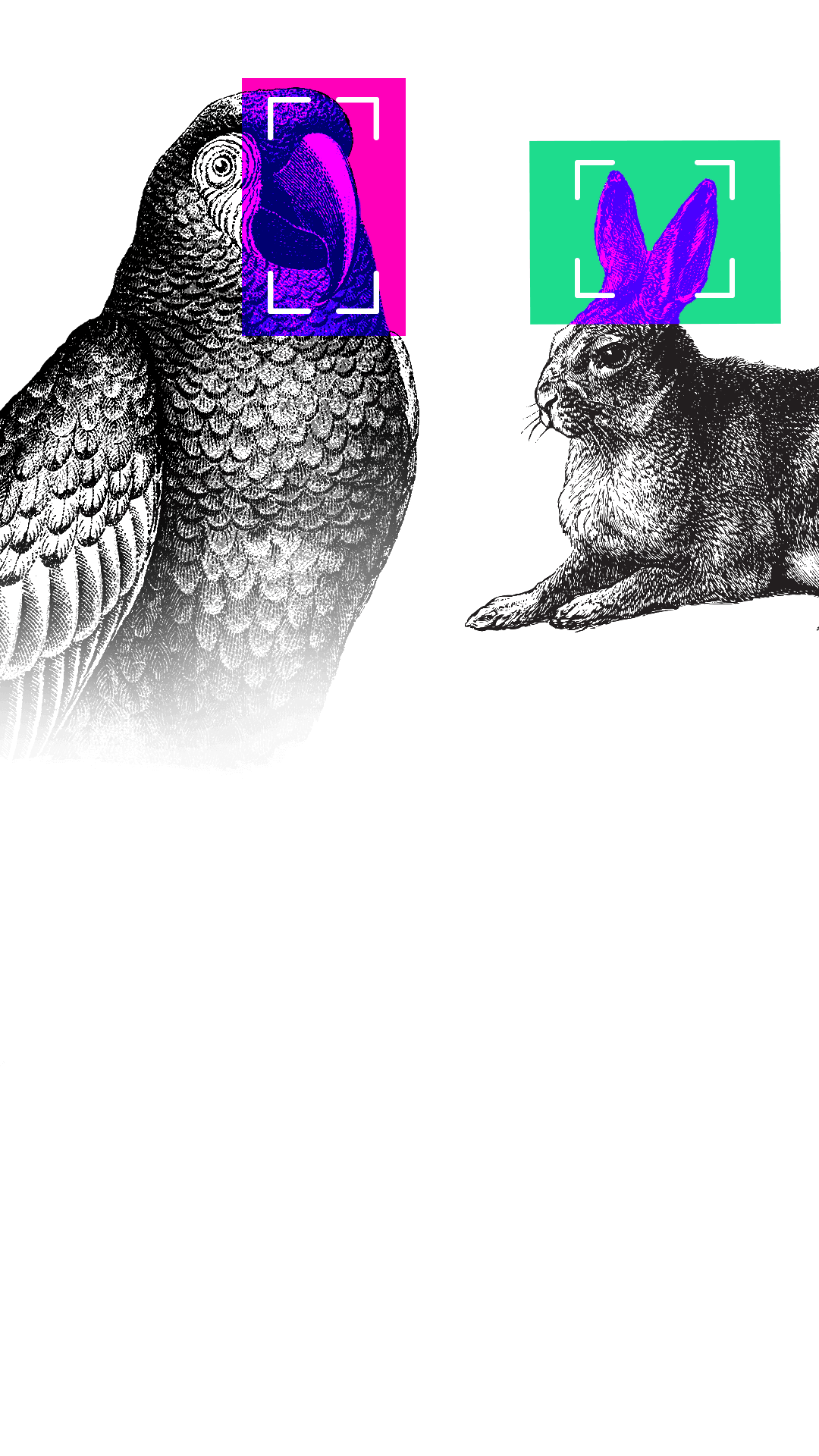
Have you got your head around artificial intelligence yet?
In the past six months, chatbots, like ChatGPT, and image generators, such as Midjourney, have rapidly become a cultural phenomenon.
But artificial intelligence (AI) or "machine learning" models have been evolving for a while.
In this beginner's guide, we'll venture beyond chatbots to discover various species of AI - and see how these strange new digital creatures are already playing a part in our lives.
How does AI learn?
The key to all machine learning is a process called training, where a computer program is given a large amount of data - sometimes with labels explaining what the data is - and a set of instructions.
The instruction might be something like: "find all the images containing faces" or, "categorise these sounds".
The program will then search for patterns in the data it has been given to achieve these goals.
It might need some nudging along the way - such as "that’s not a face" or "those two sounds are different" - but what the program learns from the data and the clues it is given becomes the AI model - and the training material ends up defining its abilities.
One way to look at how this training process could create different types of AI is to think about different animals.
Over millions of years, the natural environment has led to animals developing specific abilities, in a similar way, the millions of cycles an AI makes through its training data will shape the way it develops and lead to specialist AI models.
So what are some examples of how we have trained AIs to develop different skills?
What are chatbots?
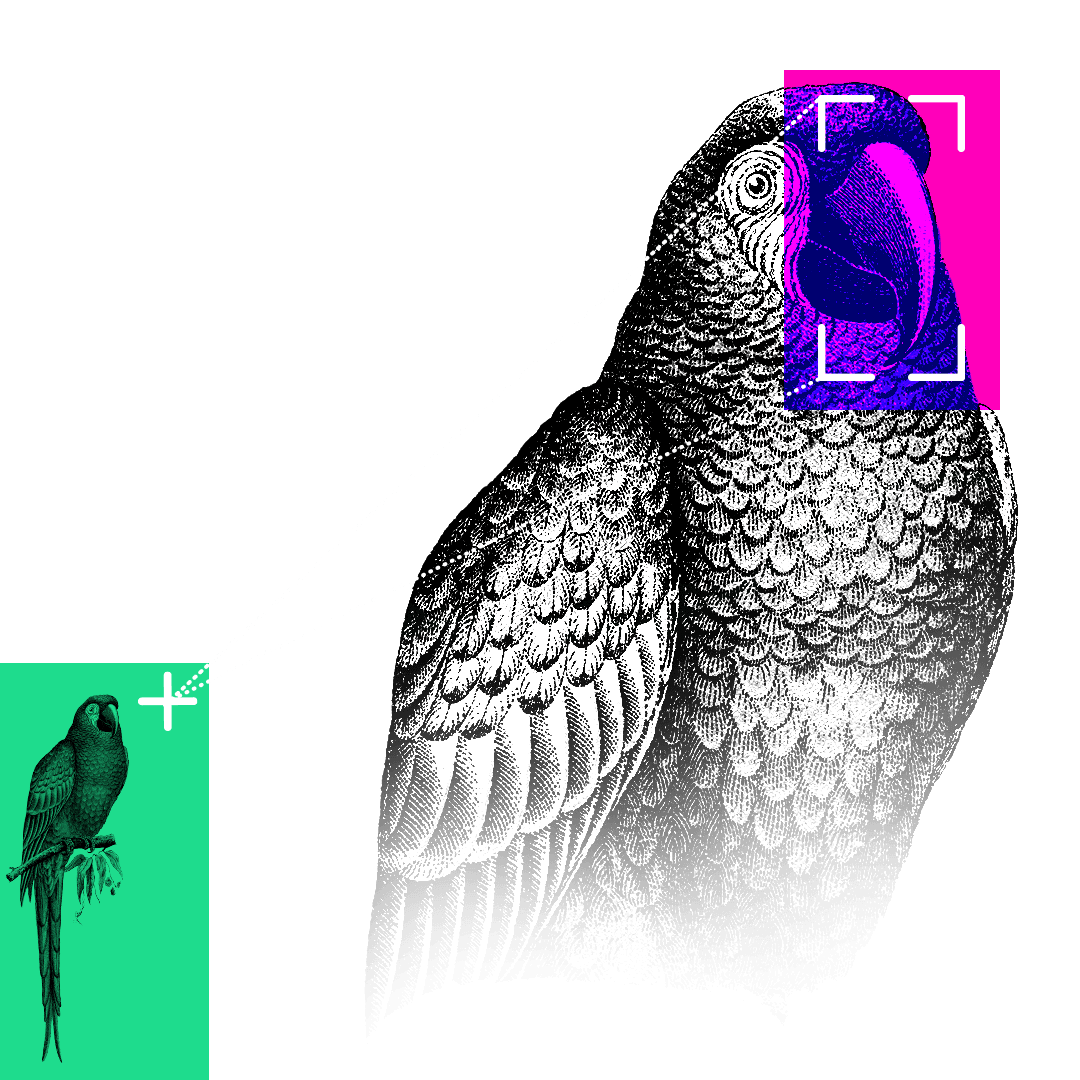
Think of a chatbot as a bit like a parrot. It’s a mimic and can repeat words it has heard with some understanding of their context but without a full sense of their meaning.
Chatbots do the same - though on a more sophisticated level - and are on the verge of changing our relationship with the written word.
But how do these chatbots know how to write?
They are a type of AI known as large language models (LLMs) and are trained with huge volumes of text.
An LLM is able to consider not just individual words but whole sentences and compare the use of words and phrases in a passage to other examples across all of its training data.
Using these billions of comparisons between words and phrases it is able to read a question and generate an answer - like predictive text messaging on your phone but on a massive scale.
The amazing thing about large language models is they can learn the rules of grammar and how to use words in the correct context, without human assistance.
Can I talk with an AI?
If you've used Alexa, Siri or any other type of voice recognition system, then you've been using AI.
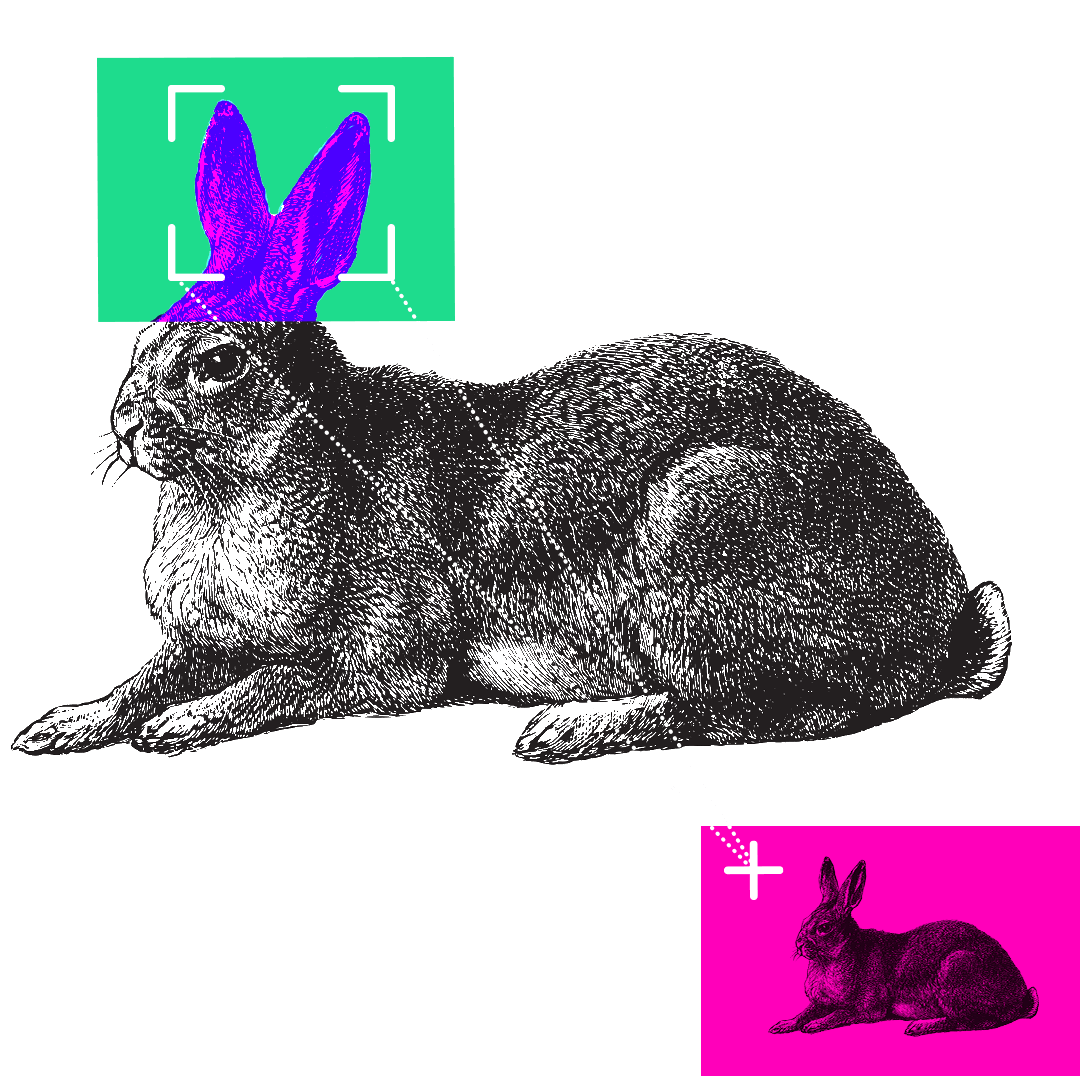
Imagine a rabbit with its big ears, adapted to capture tiny variations in sound.
The AI records the sounds as you speak, removes the background noise, separates your speech into phonetic units - the individual sounds that make up a spoken word - and then matches them to a library of language sounds.
Your speech is then turned into text where any listening errors can be corrected before a response is given.
This type of artificial intelligence is known as natural language processing.
It is the technology behind everything from you saying "yes" to confirm a phone-banking transaction, to asking your mobile phone to tell you about the weather for the next few days in a city you are travelling to.
Can AI understand images?
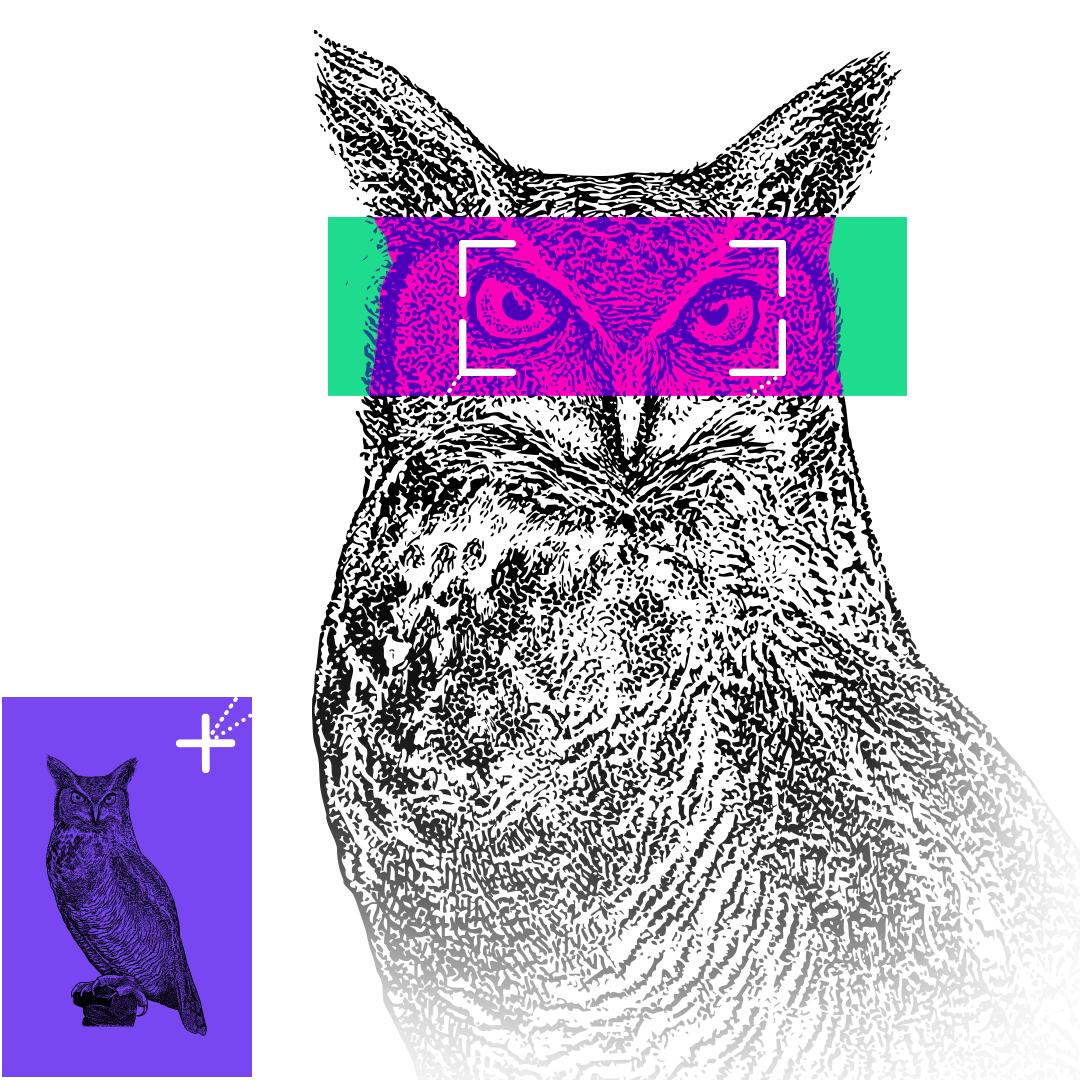
Has your phone ever gathered your photos into folders with names like "at the beach" or "nights out"?
Then you’ve been using AI without realising. An AI algorithm uncovered patterns in your photos and grouped them for you.
These programs have been trained by looking through a mountain of images, all labelled with a simple description.
If you give an image-recognition AI enough images labelled "bicycle", eventually it will start to work out what a bicycle looks like and how it is different from a boat or a car.
Sometimes the AI is trained to uncover tiny differences within similar images.
This is how facial recognition works, finding a subtle relationship between features on your face that make it distinct and unique when compared to every other face on the planet.
The same kind of algorithms have been trained with medical scans to identify life-threatening tumours and can work through thousands of scans in the time it would take a consultant to make a decision on just one.
How does AI make new images?
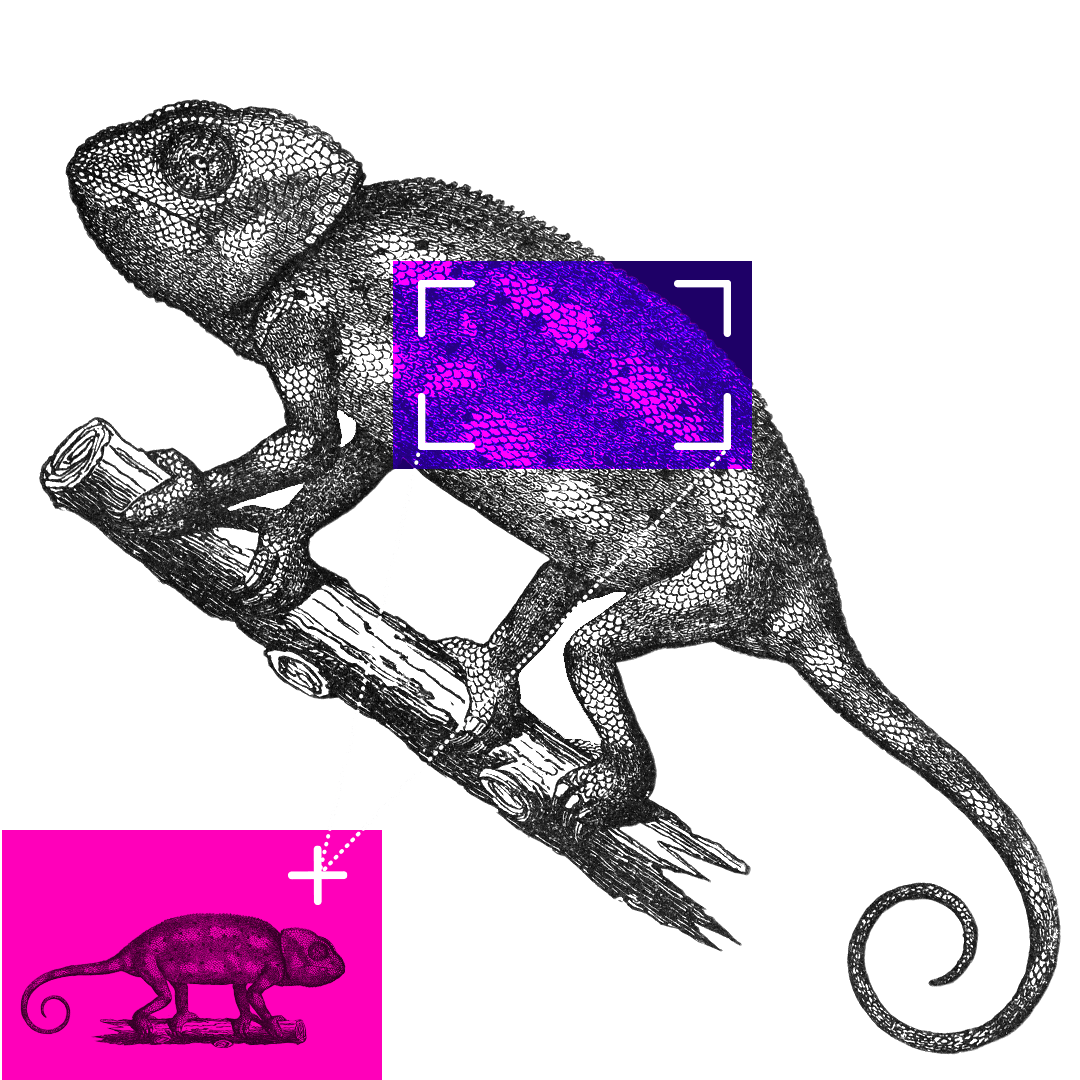
Recently image recognition has been adapted into AI models which have learned the chameleon-like power of manipulating patterns and colours.
These image-generating AIs can turn the complex visual patterns they gather from millions of photographs and drawings into completely new images.
You can ask the AI to create a photographic image of something that never happened - for example, a photo of a person walking on the surface of Mars.
Or you can creatively direct the style of an image: "Make a portrait of the England football manager, painted in the style of Picasso."
The latest AIs start the process of generating this new image with a collection of randomly coloured pixels.
It looks at the random dots for any hint of a pattern it learned during training - patterns for building different objects.
These patterns are slowly enhanced by adding further layers of random dots, keeping dots which develop the pattern and discarding others, until finally a likeness emerges.
Develop all the necessary patterns like "Mars surface", "astronaut" and "walking" together and you have a new image.
Because the new image is built from layers of random pixels, the result is something which has never existed before but is still based on the billions of patterns it learned from the original training images.
Society is now beginning to grapple with what this means for things like copyright and the ethics of creating artworks trained on the hard work of real artists, designers and photographers.
What about self-driving cars?
Self-driving cars have been part of the conversation around AI for decades and science fiction has fixed them in the popular imagination.
Self-driving AI is known as autonomous driving and the cars are fitted with cameras, radar and range-sensing lasers.
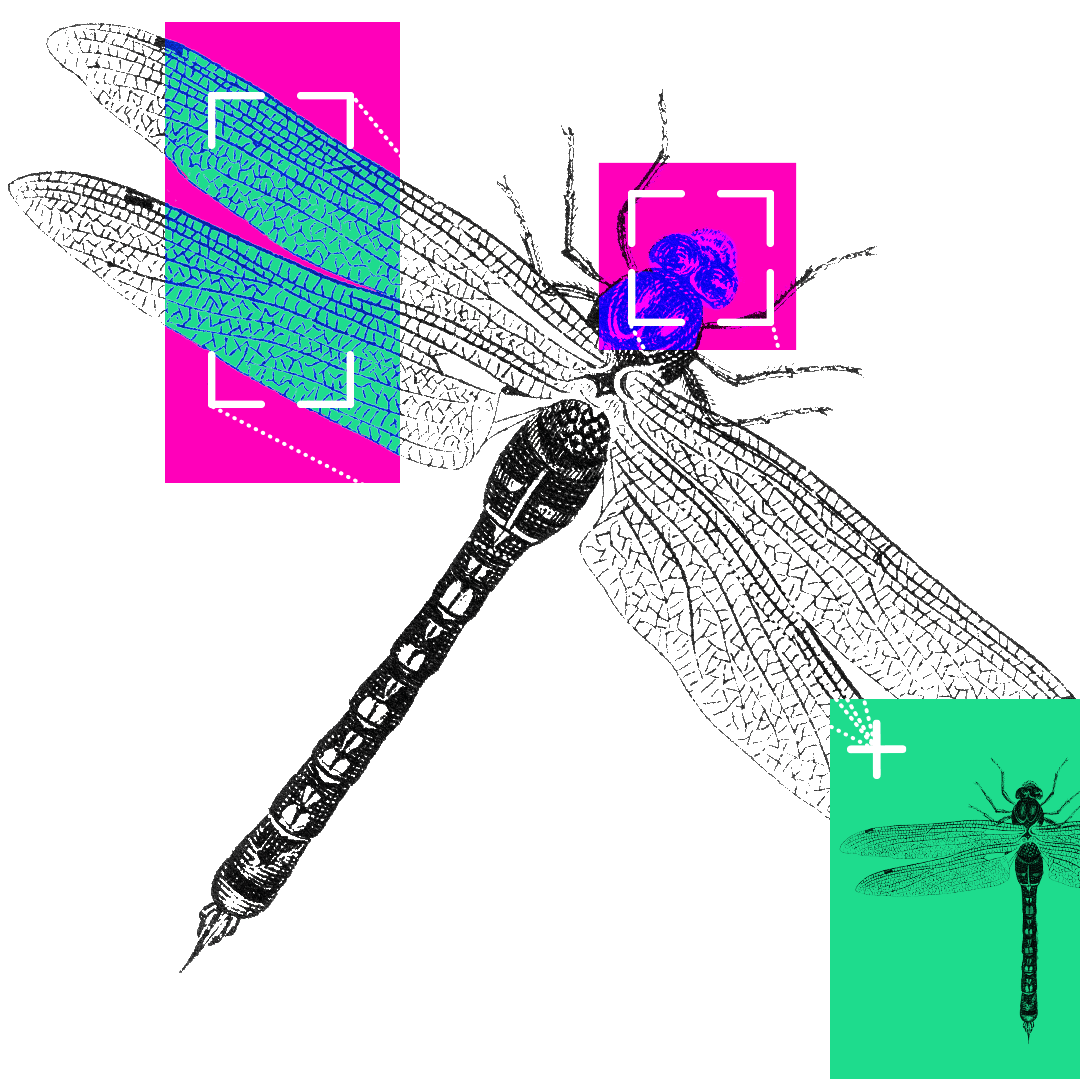
Think of a dragonfly, with 360-degree vision and sensors on its wings to help it manoeuvre and make constant in-flight adjustments.
In a similar way, the AI model uses the data from its sensors to identify objects and figure out whether they are moving and, if so, what kind of moving object they are - another car, a bicycle, a pedestrian or something else.
Thousands and thousands of hours of training to understand what good driving looks like has enabled AI to be able to make decisions and take action in the real world to drive the car and avoid collisions.
Predictive algorithms may have struggled for many years to deal with the often unpredictable nature of human drivers, but driverless cars have now collected millions of miles of data on real roads. In San Francisco, they are already carrying paying passengers.
Autonomous driving is also a very public example of how new technologies must overcome more than just technical hurdles.
Government legislation and safety regulations, along with a deep sense of anxiety over what happens when we hand over control to machines, are all still potential roadblocks for a fully automated future on our roads.
What does AI know about me?
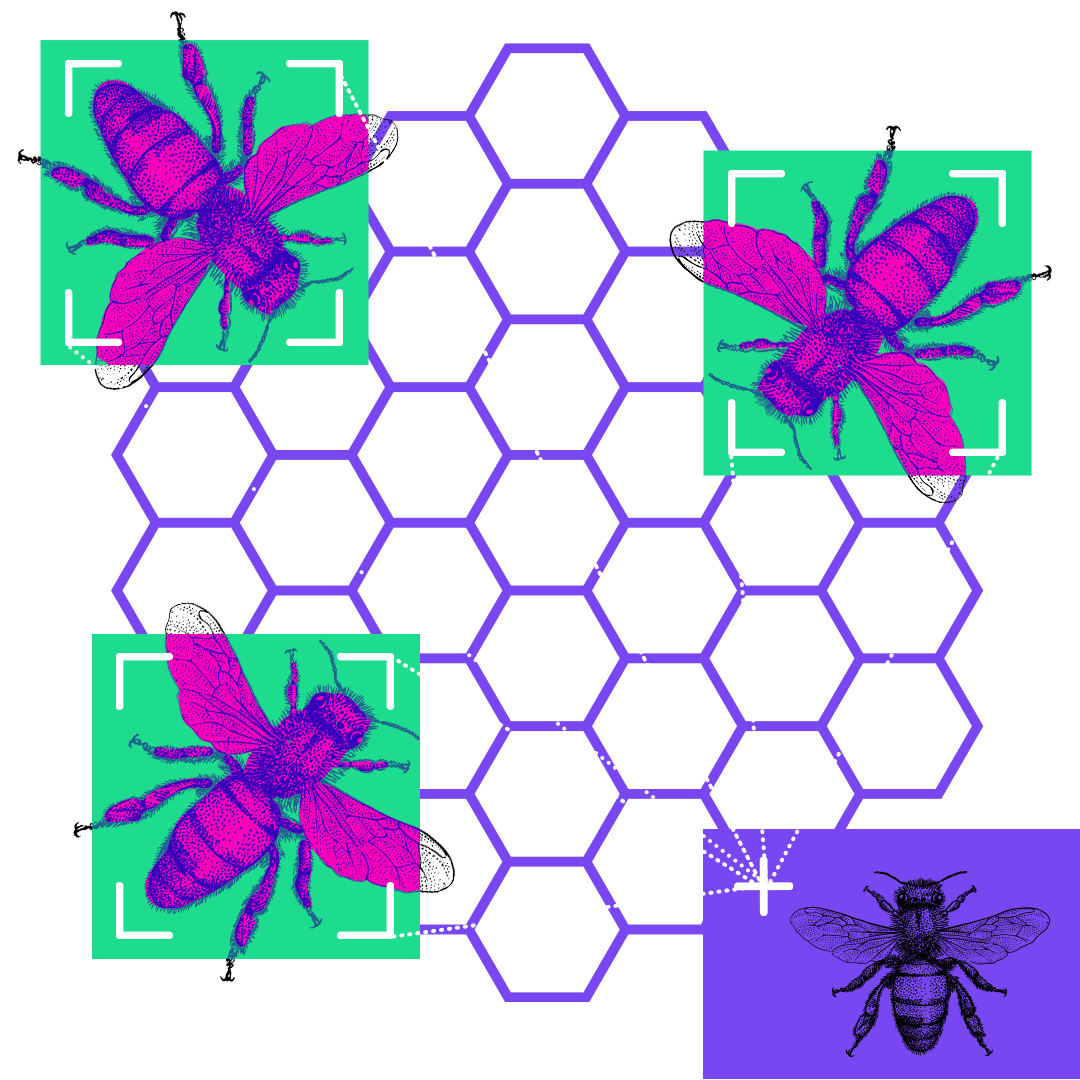
Some AIs simply deal with numbers, collecting and combining them in volume to create a swarm of information, the products of which can be extremely valuable.
There are likely already several profiles of your financial and social actions, particularly those online, which could be used to make predictions about your behaviour.
Your supermarket loyalty card is tracking your habits and tastes through your weekly shop. The credit agencies track how much you have in the bank and owe on your credit cards.
Netflix and Amazon are keeping track of how many hours of content you streamed last night. Your social media accounts know how many videos you commented on today.
And it’s not just you, these numbers exist for everyone, enabling AI models to churn through them looking for social trends.
These AI models are already shaping your life, from helping decide if you can get a loan or mortgage, to influencing what you buy by choosing which ads you see online.
Will AI be able to do everything?
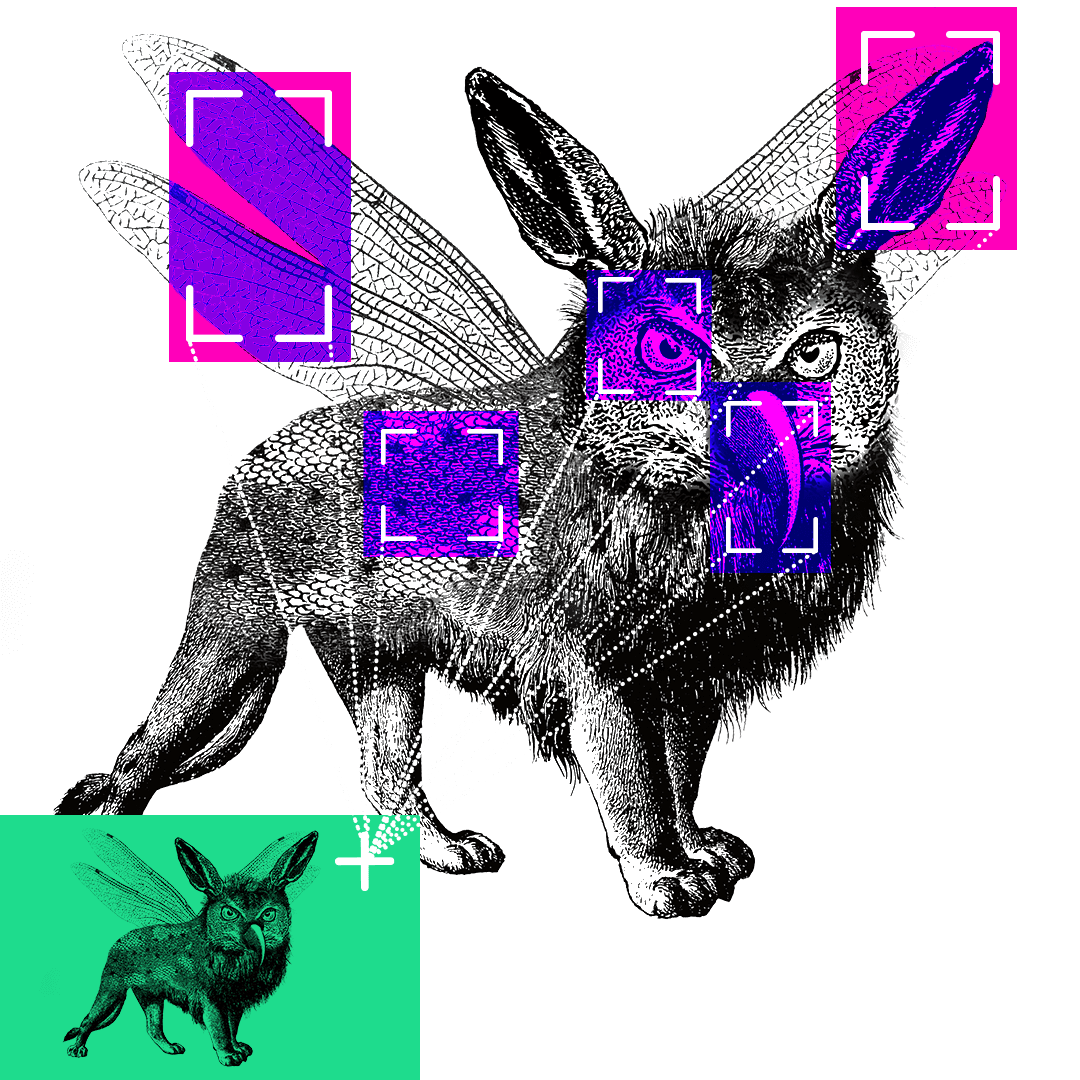
Would it be possible to combine some of these skills into a single, hybrid AI model?
That is exactly what one of the most recent advances in AI does.
It’s called multimodal AI and allows a model to look at different types of data - such as images, text, audio or video - and uncover new patterns between them.
This multimodal approach was one of the reasons for the huge leap in ability shown by ChatGPT when its AI model was updated from GPT3.5, which was trained only on text, to GPT4, which was trained with images as well.
The idea of a single AI model able to process any kind of data and therefore perform any task, from translating between languages to designing new drugs, is known as artificial general intelligence (AGI).
For some it’s the ultimate aim of all artificial intelligence research; for others it’s a pathway to all those science fiction dystopias in which we unleash an intelligence so far beyond our understanding that we are no longer able to control it.
How do you train an AI?
Until recently the key process in training most AIs was known as "supervised learning".
Huge sets of training data were given labels by humans and the AI was asked to figure out patterns in the data.
The AI was then asked to apply these patterns to some new data and give feedback on its accuracy.
For example, imagine giving an AI a dozen photos - six are labelled "car" and six are labelled "van".
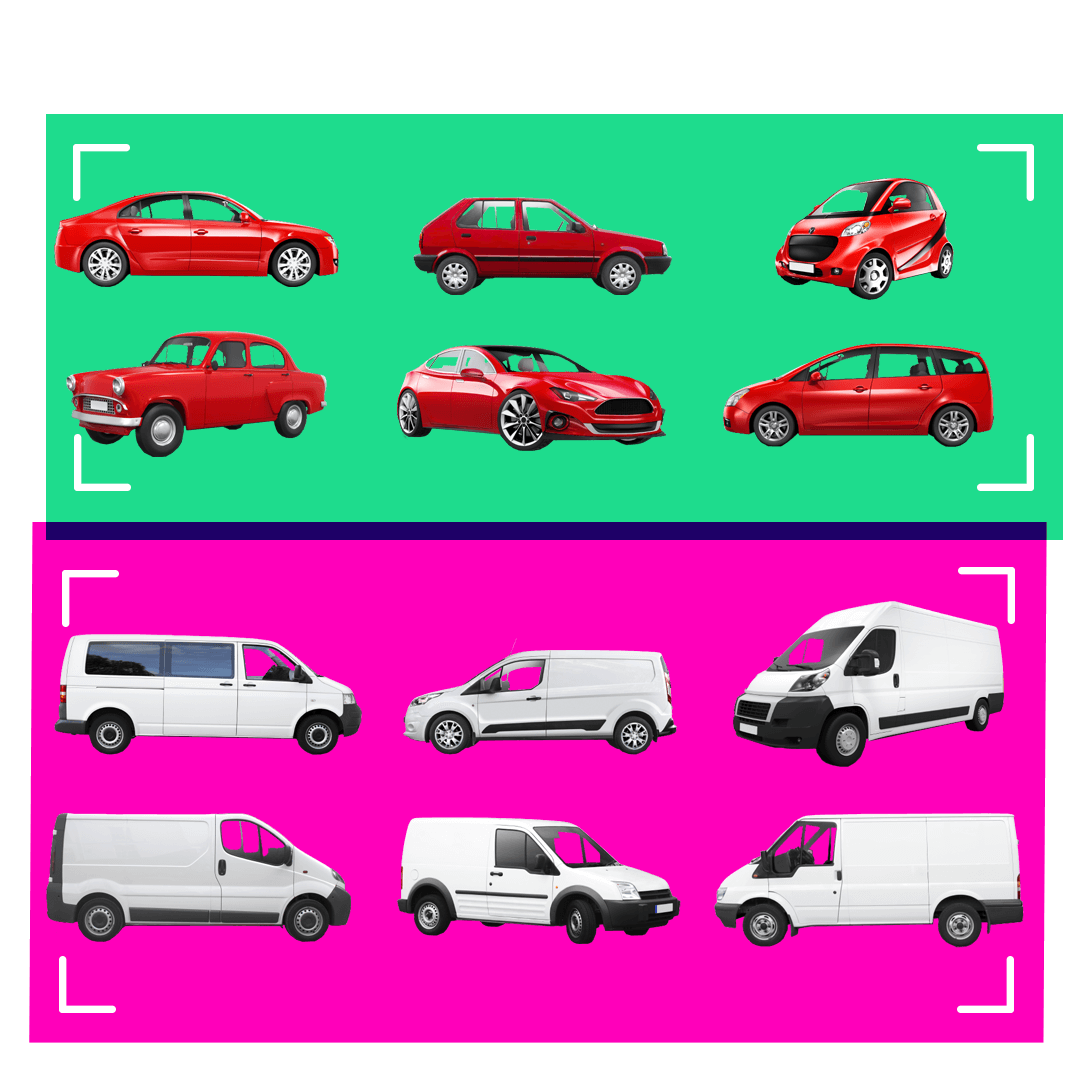
Next tell the AI to work out a visual pattern that sorts the cars and the vans into two groups.
Now what do you think happens when you ask it to categorise this photo?
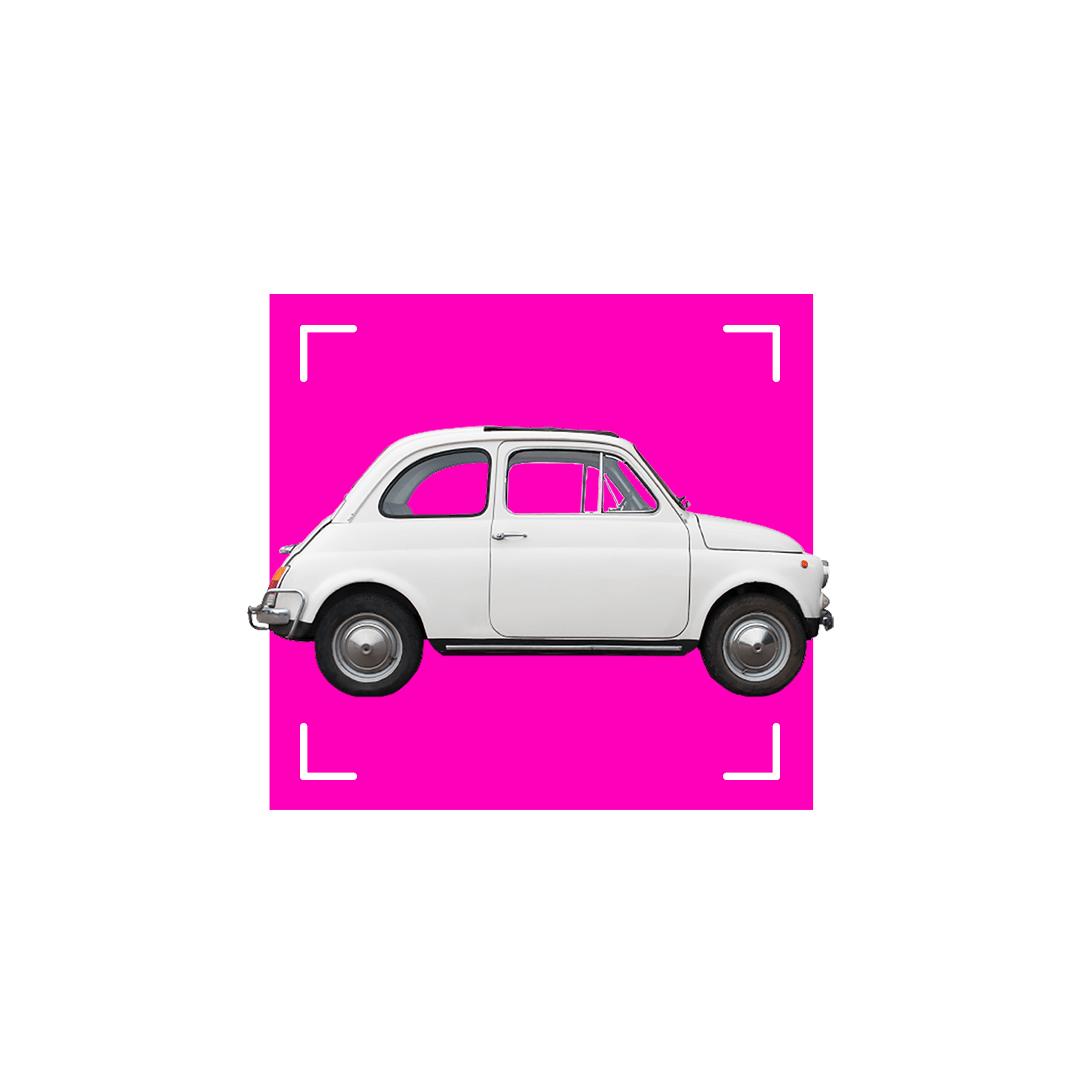
Unfortunately, it seems the AI thinks this is a van - not so intelligent.
Now you show it this.
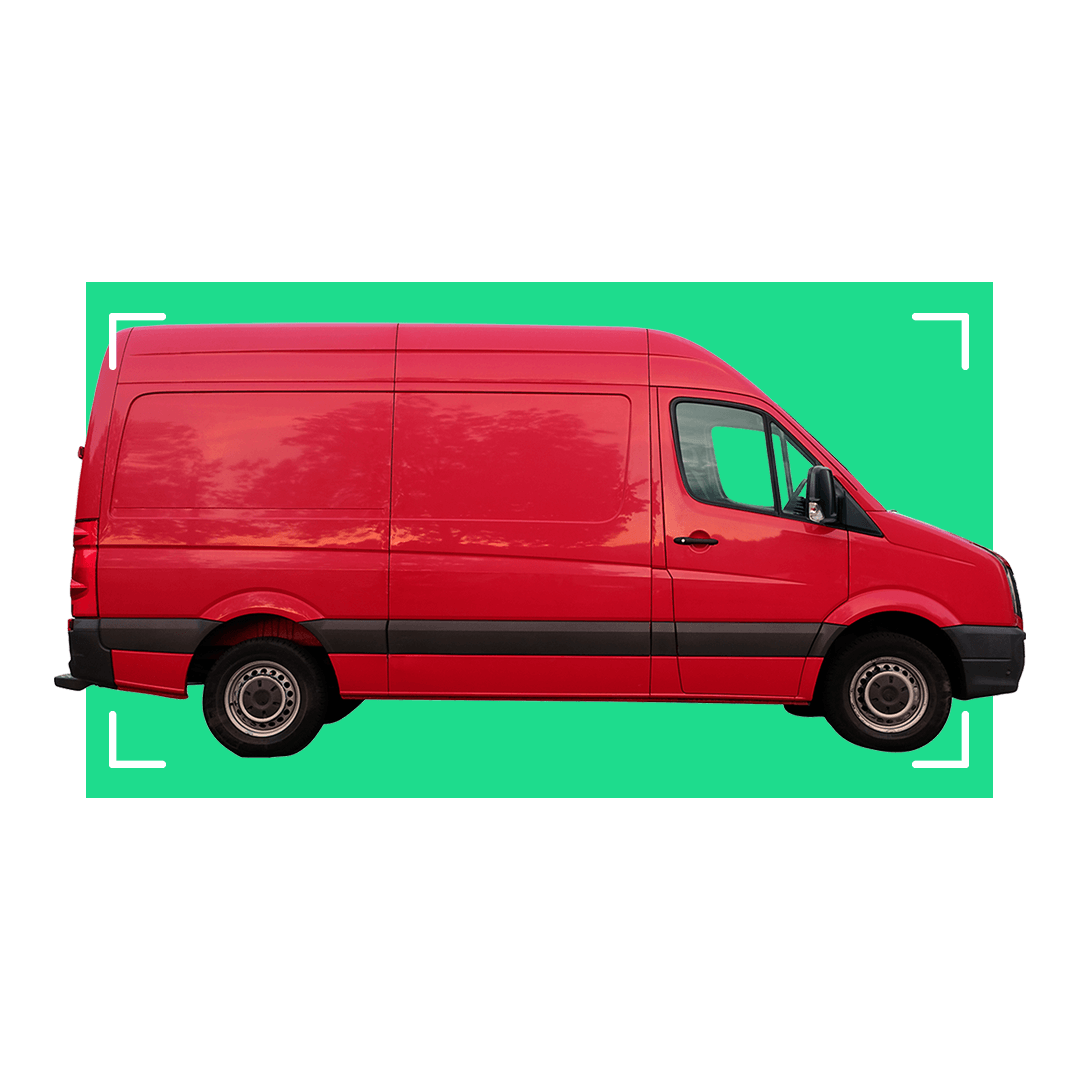
And it tells you this is a car.
It’s pretty clear what’s gone wrong.
From the limited number of images it was trained with, the AI has decided colour is the strongest way to separate cars and vans.
But the amazing thing about the AI program is that it came to this decision on its own - and we can help it refine its decision-making.
We can tell it that it has wrongly identified the two new objects - this will force it to find a new pattern in the images.
But more importantly, we can correct the bias in our training data by giving it more varied images.
These two simple actions taken together - and on a vast scale - are how most AI systems have been trained to make incredibly complex decisions.
How does AI learn on its own?
Supervised learning is an incredibly powerful training method, but many recent breakthroughs in AI have been made possible by unsupervised learning.
In the simplest terms, this is where the use of complex algorithms and huge datasets means the AI can learn without any human guidance.
ChatGPT might be the most well-known example.
The amount of text on the internet and in digitised books is so vast that over many months ChatGPT was able to learn how to combine words in a meaningful way by itself, with humans then helping to fine-tune its responses.
Imagine you had a big pile of books in a foreign language, maybe some of them with images.
Eventually you might work out that the same word appeared on a page whenever there was drawing or photo of a tree, and another word when there was a photo of a house.
And you would see that there was often a word near those words that might mean “a” or maybe “the” - and so on.
ChatGPT made this kind of close analysis of the relationship between words to build a huge statistical model which it can then use to make predictions and generate new sentences.
It relies on enormous amounts of computing power which allows the AI to memorise vast amounts of words - alone, in groups, in sentences and across pages - and then read and compare how they are used over and over and over again in a fraction of a second.
Should I be worried about AI?
The rapid advances made by deep learning models in the last year have driven a wave of enthusiasm and also led to more public engagement with concerns over the future of artificial intelligence.
There has been much discussion about the way biases in training data collected from the internet – such as racist, sexist and violent speech or narrow cultural perspectives - leads to artificial intelligence replicating human prejudices.
Another worry is that artificial intelligence could be tasked to solve problems without fully considering the ethics or wider implications of its actions, creating new problems in the process.
Within AI circles this has become known as the "paperclip maximiser problem”, after a thought experiment by the philosopher Nick Bostrom.
He imagined an artificial intelligence asked to create as many paperclips as possible which slowly diverts every natural resource on the planet to fulfil its mission – including killing humans to use as raw materials for more paperclips.
Others say that, rather than focusing on murderous AIs of the future, we should be more concerned with the immediate problem of how people could use existing AI tools to increase distrust in politics and scepticism of all forms of media.
In particular, the world's eyes are on the 2024 presidential election in the US, to see how voters and political parties cope with a new level of sophisticated disinformation.
What happens if social media is flooded with fake videos of presidential candidates, created with AI and each tailored to anger a different group of voters?
In Europe, the EU is creating an Artificial Intelligence Act to protect its citizens' rights by regulating the deployment of AI – for instance, a ban on using facial recognition to track or identify people in real-time in public spaces.
These are among the first laws in the world to establish guidelines for the future use of these technologies – setting boundaries on what companies and governments will and will not be allowed to do – but, as the capabilities of artificial intelligence continue to grow, they are unlikely to be the last.